Introduction to AI Demand Forecasting: Benefits & Best Practices
Introduction to AI Demand Forecasting: Benefits & Best Practices
Knowing what’s coming can help you prepare. Artificial intelligence (AI) demand forecasting can enable businesses to meet customer expectations, keep up with the changing market, and cut down on excess inventory. We’ll talk about the role AI demand forecasting can play, its benefits, and best practices to get the most out of the technology.
What is AI Demand Forecasting?
AI demand forecasting leverages predictive artificial intelligence to estimate future demand for services or products based on historical data, external market factors, consumer buying patterns, and more.
How Does AI Demand Forecasting Work?
Demand forecasting can be done manually by analyzing various internal and external data sets to make both short- and long-term predictions, but AI models can greatly improve the process. Humans can only take in and make sense of so much data, whereas AI can sift through more information faster and use machine learning (ML) algorithms to more closely examine trends and patterns that may otherwise go ignored. AI then actively learns from historical data, adapts to changing trends, and improves accuracy over time through iterative training processes.
AI can take a few different approaches to demanding forecasting, such as:
- Time Series Analysis: A statistical technique that uses past sales data to uncover patterns, trends, and seasonality to help forecast demand.
- Neural Networks: Analyzes sales data, along with other factors, to predict demand and can utilize deep learning to capture and uncover complex patterns within the data.
- Ensemble Methods: This approach combines multiple models to lessen the weaknesses of individual models and improves overall prediction accuracy.
6 Categories of AI Used in Demand Forecasting
Depending on the type of planning being done, one or more of the six types of AI may be used in demand forecasting.
- Short-Term Demand Forecasting: Inventory management and production planning can benefit from relatively short-term horizon, which can predict what demand may look like typically within a window of about 12 months or less.
- Long-Term Demand Forecasting: For longer time frames, long-term AI forecasting can be used, which can generally predict up to around 4 years. This is helpful for strategic planning, significant investments, and supply chain management.
- Active AI Forecasting: AI techniques used for predicting the outcome or performance of specific campaigns, growth plans, or business initiatives in real-time. This is particularly useful for startups and businesses seeking funding, because it provides insights into potential growth trajectories.
- Passive Forecasting: By looking into historical data through AI to forecast future operations, financials, or demand trends without real-time intervention. This can help businesses plan their strategies and resource allocation effectively.
- Internal Data Utilization: Understanding starts at a business’s front door, gathering organizational data such as sales records, operational metrics, and customer data, and using AI techniques to generate insights about services, products, channels, and locations within the business.
- External Data Utilization: If organizations don’t have internal data, or they’re looking for another perspective on a situation, they can use external information from competitors and overall trends in the market and apply AI techniques to gain additional perspectives on market dynamics to make informed decisions about future directions.
Benefits of AI Demand Forecasting
AI demand forecasting can help your business automate workflows, achieve better accuracy, limit your risk, and improve customer satisfaction.
Workflow Automation
If you’re analyzing demand manually, you might not see patterns that allow you to respond in a timely manner. Automating data collection and analysis can improve the forecasting process. You may even be able to form real-time insights that lead to faster and more authoritative decision-making. You’ll also leave less up to chance and reduce errors in data analysis compared to manual calculation.
Keep in mind that automating workflows not only expedites the decision-making process, but also fosters scalability and resource optimization within an organization. By eliminating manual data entry and analysis tasks, AI streamlines workflows and allows teams to focus on more strategic projects vs mundane operational activities. The added scalability also enhances operational efficiency and can decrease overhead costs associated with manual labor.
Plus, as a business grows, AI-driven automation adapts seamlessly and continues to ensure consistent performance and accurate insights.
Better Accuracy and Efficiency
Accuracy isn’t just improved by automating workflows. Artificial intelligence models can take massive amounts of data and surface trends humans might miss, boosting the accuracy of forecasts. With greater accuracy, resources can be placed more effectively based on demand, and businesses can achieve a balance between overstocking and selling out. Additionally, improved accuracy leads to better resource allocation and inventory management, ultimately driving efficiency and cost savings.
Risk Mitigation Through Planning
When you’re able to see changes in demand, you can also foresee potential risks, including supply chain disruptions or emerging market shifts that may impact your business that either drives or runs concurrently with demand. Better business planning can also maximize business continuity.
Enhanced Customer Satisfaction
When customers can get what they need, when they need it, through demand forecasting and business continuity measures, they will experience fewer disappointments due to out-of-stock items. You can meet and even exceed customer demand expectations by suggesting products and promotions based on demand patterns of certain customer profiles. These personalized recommendations and timely inventory management also contribute to a positive customer experience, fostering loyalty and retention.
Companies can gain a competitive edge over other businesses in their industry when AI demand forecasting is used to its fullest potential, particularly with edge computing, which can bring computing power closer to the end user and maximize performance.
Challenges of AI Demand Forecasting
While AI can offer unique benefits to businesses looking to meet demand and surpass customer expectations, implementing new technology also comes with challenges.
Data Dependency and Quality
Your forecasts are only as reliable as the data at your disposal. If the data you have isn’t robust, or is low-quality in another way, your forecasts can also suffer. Sometimes, data may require cleaning and preparation before it’s ready for in-depth insights, or the set you have may be biased or skewed in a way that’s not immediately apparent.
Unforeseen Patterns
While AI models can do a great job of identifying patterns from historical data, they may have a harder time identifying new shifts that are indicative of emerging trends. It’s important not to rely solely on the human eye or an AI algorithm to make decisions about demand, but instead, rely on a combination of the two. It can also be difficult to determine how AI made a particular decision about demand due to the black box nature of some AI models.
Continuous Maintenance
Even though AI can cut down on human intervention and save time long-term, when new AI models are being used, they will require a lot of maintenance and attention to fine-tune and become useful. Your team needs to choose the right algorithm, make updates to the model, and monitor performance to solve any issues that arise and course-correct as needed.
Integration
Artificial intelligence models generally exhibit better performance when operating within cloud computing environments, but integration can still be a concern for businesses that have come far in their digital transformation journeys. It’s even harder to integrate with legacy frameworks. Some AI tools and platforms may not even be compatible with each other. Understanding the capabilities of demand forecasting tools and their ability to integrate with other tools already in your organization’s tech stack will make for a smoother implementation.
Lack of Customization
Unless you have someone on your team who can customize an AI model, you may be left to use off-the-shelf tools that don’t have all the ideal features you’d like to see. Even if you have the capabilities to build from scratch, the process can be time-consuming and expensive. Your business will eventually have to decide between having the flexibility to customize the tool to fit your needs and compromising in the name of time and budget.
Use Cases for AI Demand Forecasting
While AI demand forecasting is primarily used for optimizing supply chain management and assisting with inventory planning, it can also be used in many other contexts for businesses looking to understand the (sometimes) hidden patterns governing their business cycles.
Developing a Product-Mix Plan
Businesses can use AI to analyze market gaps, peak purchase times, and product visibility. These data points can aid in deciding when to roll out new products, what needs to be retired, and what quantities to order without over- or under-estimating supply.
Forecasting Fluctuating Purchase Patterns
Buying patterns are more likely to be cyclical than linear. AI can help plan for seasonal product demand and predict supply chain costs by identifying fluctuating patterns and optimizing sales and operations planning accordingly.
Minimizing Overstock and Stockouts
When you can automatically analyze all available data and constraints through AI solutions, you can also constantly rebalance supply and demand and ensure the right amount of product is ordered at the right times. You can get much closer to “just perfect” inventory levels, while avoiding overstock and stockout situations.
Predicting Website Traffic
Having the right inventory for demand surges doesn’t mean anything if your website can’t handle the influx of traffic. AI can also be used to predict these traffic increases and allocate resources to accommodate bursts in demand from surprise sales or seasonal events.
Workflow Automation and Data Consolidation
When configured properly, AI solutions can integrate with all data sources. This enables the data in an ERP, for example, to be updated automatically through the submission of daily suggested transfer and manufacturing orders to the system. Workflow automation measures can streamline demand planning and eliminate manual processes from routine activities.
Best Practices for Implementing AI Demand Forecasting
Demand forecasting is meant to solve a problem, but first, you need to be clear on your questions and objectives. Here are some best practices for implementing AI demand forecasting at your organization.
1. Defining the Forecasting Problem and Objectives
What are you trying to achieve? Do you want to optimize your inventory levels, refine your supply chain, or personalize marketing campaigns for certain customer profiles? Demand forecasting can help you accomplish these goals, but you must define what you’re looking for first.
2. Use an AI Adoption Framework
When embracing AI, starting from square one isn’t always necessary. Utilizing various Ai adoption frameworks like the Google Cloud AI Adoption Framework, Gartner Hype Cycle, or Microsoft’s AI Adoption Framework can guide you through the process, ensuring the integration and transition to AI technologies is easy and successful.
3. Data Collection and Preparation
Be sure that the data you are collecting is relevant, high-quality, and prepared properly. The better you are sourcing your data from internal and external sources, the easier it will be to make well-informed insights.
4. Selecting the Right AI Tools and Platforms
As we’ve mentioned, selecting the right AI tools and platforms has to do with the functionality you’re looking for, the services you need, and the integrations necessary to get everything working together. It’s important to work with specialized consultants who can ensure the right tools and platforms are selected based on your goals, such as scalability, security, and vendor support.
5. Incorporating Machine Learning
ML algorithms may be part of the AI tools you’ve chosen or may be used in conjunction with data you’re gathering, to help with the demand forecasting process. Linear regression can uncover a linear relationship between two sets of data. Support vector machines can divide data points based on high and low demand. Neural networks can form connections much like the human brain between points that are non-linear and may not seem related at first.
6. Training Users and Managing Data Quality
AI models can get you far, but you need to have human capabilities to take generate action based on your insights. Train users on how these new demand forecasting tools work, and how to refine the models for greater accuracy and quality as time goes on.
Empowering Your Business with AI Demand Forecasting
Leave guesswork in the past. AI demand forecasting can help businesses become more proactive and data-driven in a way that was not previously possible. If you’re interested in learning how AI can transform your business, download the Business Applications of AI/ML whitepaper and contact us to discover how TierPoint’s AI consulting services can help you unlock these capabilities.
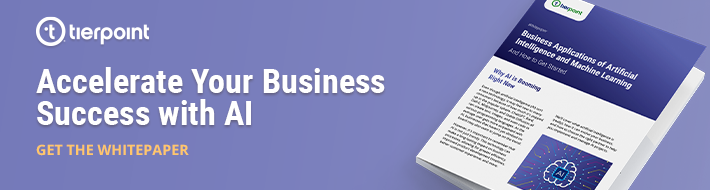
FAQs
AI can shift the demand forecasting process from reactive to proactive, analyzing vast amounts of data to form real-time insights that allow businesses to meet demand more efficiently and accurately compared to manual methods.
Generative AI can’t really be used for forecasting. It’s more for creative projects and ideation and less about data modeling to form predictions.
An example of AI demand forecasting could be a company using historical data to determine when their peak sales period will be, and which product inventories they will need to increase to meet this peak sales time.
More >> Introduction to AI Demand Forecasting: Benefits & Best Practices